Data Labeling: The Backbone of AI and Machine Learning
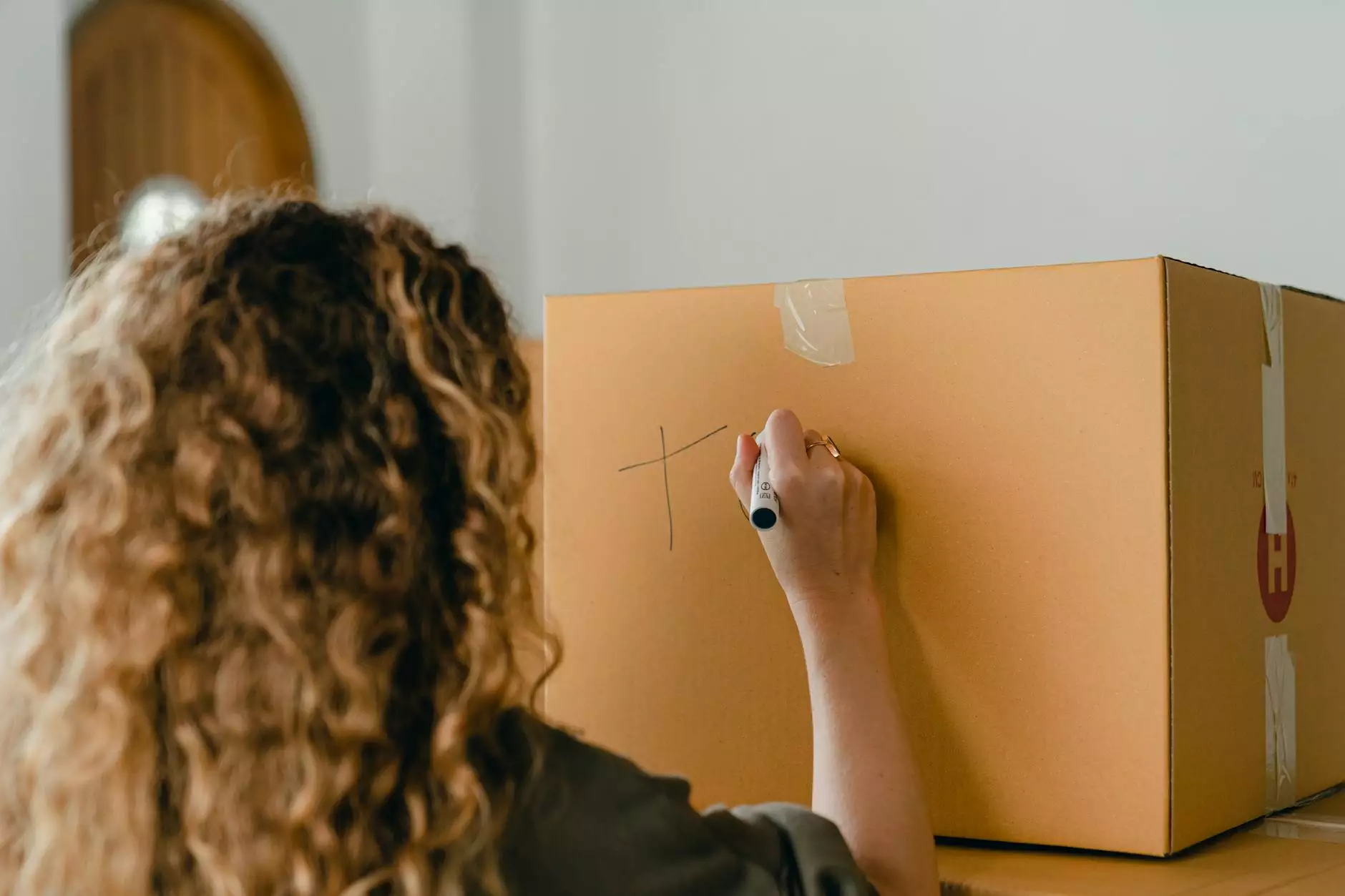
Data labeling is an essential process that enables artificial intelligence (AI) and machine learning (ML) models to understand and interpret the world. As businesses across various industries seek to harness the power of AI, the importance of meticulously labeled data cannot be overstated. In this article, we will explore the concept of data labeling, its significance in the realm of artificial intelligence, and how platforms like Keylabs.ai can revolutionize your data annotation processes.
What is Data Labeling?
Data labeling involves the process of tagging, annotating, or categorizing data so that machine learning algorithms can learn from it. It is a crucial step in the data preparation process, where raw data is transformed into a structured format. This can include various types of data, such as images, text, audio, and video. The aim of data labeling is to provide context to the data, making it interpretable for algorithms and ensuring accurate predictions and classifications.
The Importance of Data Labeling in AI
At the core of any successful AI model lies a finely tuned dataset. Here are several reasons why data labeling is vital for AI and ML initiatives:
1. Improved Model Accuracy
A well-labeled dataset leads to higher accuracy in model predictions. When data is accurately labeled, the algorithms can learn better patterns, making them more reliable in real-world applications.
2. Enhanced Data Quality
Structured and annotated data is easier to analyze. High-quality data enables businesses to derive meaningful insights, driving better decision-making and strategic planning.
3. Facilitating Complex Problem Solving
Many AI applications, such as image recognition, natural language processing, and sentiment analysis, require extensive data labeling to function effectively. Without proper annotations, these complex tasks would be challenging for machines to tackle.
4. Training and Testing
Data labeling is essential for both training machine learning models and testing their performance. A labeled dataset allows companies to benchmark their models accurately and iterate on them based on performance metrics.
The Process of Data Labeling
Understanding the process of data labeling can help businesses set up effective annotation workflows. Here’s a step-by-step overview:
Step 1: Define the Objectives
Before starting the data labeling process, it is crucial to define the objectives. What problem are you trying to solve? What kind of model do you wish to train? These questions will guide the entire process.
Step 2: Select the Data
The next step is to gather the raw data that needs labeling. Depending on the application, this may include images, videos, speech, or written text. The quality and quantity of this raw data directly impact the efficacy of the machine learning model.
Step 3: Choose a Labeling Method
There are several methods for labeling data, including:
- Manual Labeling: Human annotators review and label the data based on predefined guidelines.
- Automated Labeling: AI-driven tools automate the labeling process using pre-trained models.
- Crowdsourced Labeling: Utilizing platforms that distribute tasks to a large number of independent annotators.
Step 4: Annotate the Data
This step involves applying the chosen labeling method to the raw data. For instance, if it involves images, annotators may draw bounding boxes around objects or classify images into specific categories.
Step 5: Quality Assurance
After labeling, it's essential to perform a quality check to ensure the accuracy of the labels. This might include random sampling and double-checking labels by different annotators to maintain a high standard.
Step 6: Finalization and Integration
Once the labeling is validated, the data is finalized and can be integrated into machine learning workflows for training models.
How Keylabs.ai Enhances Data Labeling
Keylabs.ai offers a comprehensive data annotation platform that streamlines the data labeling process. Here’s how it empowers businesses:
User-Friendly Interface
The platform provides a highly intuitive interface that simplifies the labeling process, making it accessible even for users with limited technical expertise.
Advanced Annotation Tools
Keylabs.ai includes advanced tools for annotating different data types. From segmentation tools for images to natural language processing suites for text, the platform caters to diverse annotation needs.
Quality Control Mechanisms
With built-in quality assurance features, Keylabs.ai ensures that the consistency and quality of labels meet predefined standards. This means businesses can rely on the data provided for high-stakes decision-making.
Scalability
As your data needs grow, Keylabs.ai scales with you. The platform can manage large volumes of data efficiently, making it suitable for both small businesses and large enterprises.
Support for Various Data Types
Whether you are working with images, videos, audio, or text, Keylabs.ai supports multiple data types, offering versatile solutions for various industries.
Applications of Data Labeling
Data labeling is pivotal across various industries, powering numerous applications:
1. Healthcare
In healthcare, labeled data helps in diagnostic imaging, identifying patterns in medical scans, and patient record analysis to enhance treatment efficacy.
2. Autonomous Vehicles
Data labeling assists autonomous vehicles in understanding their environment, interpreting visual data from sensors, and recognizing objects on the road.
3. E-Commerce
In the e-commerce sector, labeled data supports personalized recommendations, product categorization, and customer sentiment analysis.
4. Finance
Financial institutions utilize labeled data for fraud detection, risk assessment, and customer behavior analysis, enhancing security and operational efficiency.
5. Marketing
Marketing departments leverage labeled data for targeted advertising, market segmentation, and trend analysis to optimize campaigns and reach their audiences effectively.
Challenges in Data Labeling
While data labeling is crucial, several challenges can arise:
1. Resource-Intensiveness
The labeling process can be time-consuming and labor-intensive, especially for large datasets. This requires careful planning and resource allocation.
2. Subjectivity
Human annotators may introduce bias or inconsistency in labeling, leading to errors. Ensuring a standardized process and comprehensive guidelines is essential.
3. High Costs
Depending on the volume and complexity of the data, labeling costs can escalate, necessitating efficient workflows and potential automation.
Conclusion
In conclusion, data labeling plays a pivotal role in the success of AI and machine learning projects. With platforms like Keylabs.ai, businesses can overcome common challenges associated with data annotation, ensuring accurate and quality datasets that enhance model performance. By investing in comprehensive data labeling strategies, companies position themselves to harness the full potential of AI, driving innovation and enabling better decision-making across various sectors.
Take Action Today!
Don’t let poor data quality hold back your AI initiatives. Explore how Keylabs.ai can simplify your data labeling process and propel your business into the future of AI. Visit Keylabs.ai to learn more about our innovative data annotation solutions!